A Variational Neural Network Model of Resource-Rational Reward Encoding in Human Planning
Zhuojun Ying, Frederick Callaway, Roy Fox, Anastasia Kiyonaga, and Marcelo Mattar
47th Annual Meeting of the Cognitive Science Society (CogSci), 2025
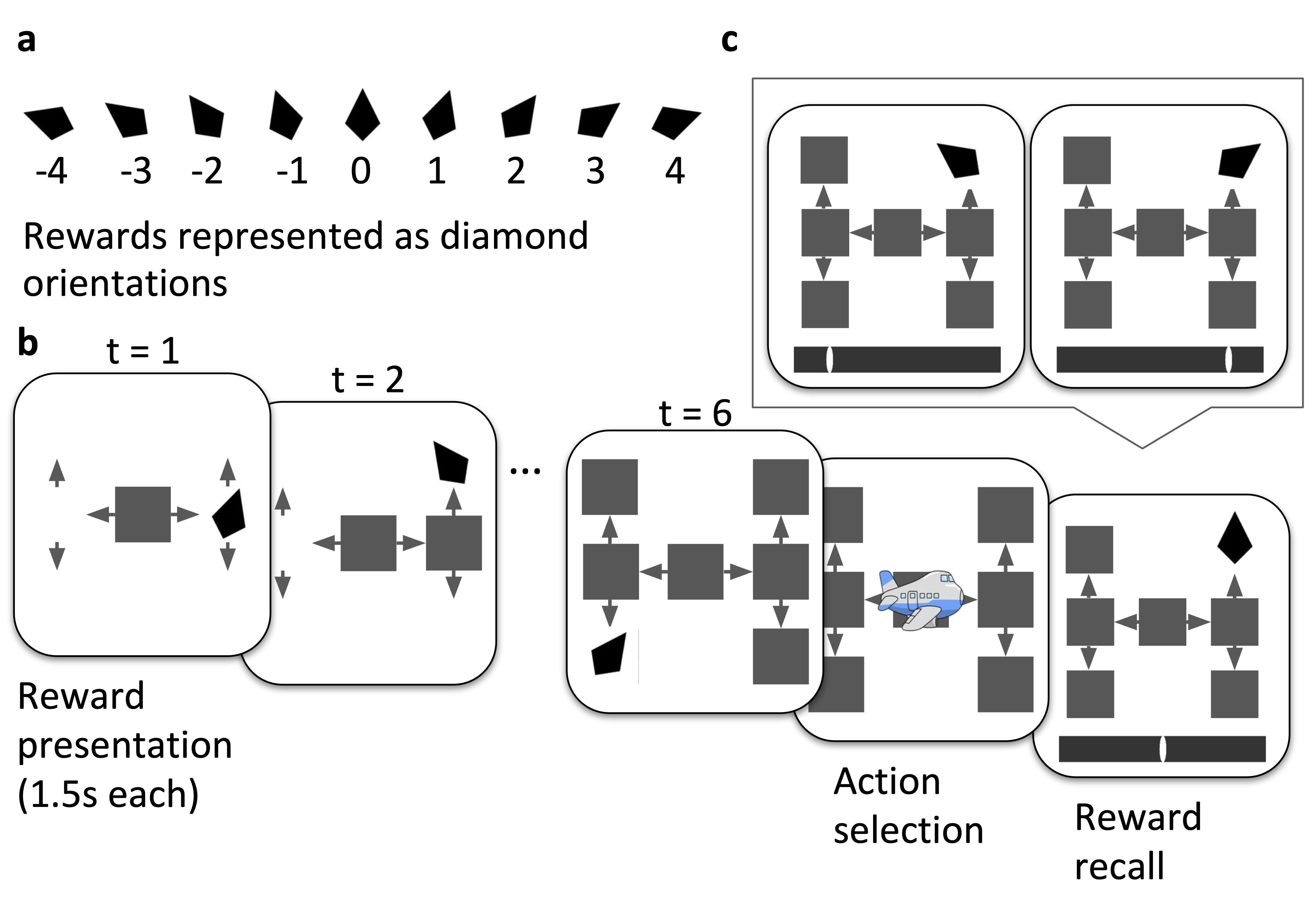
Working memory (WM) is essential for planning and decision-making, enabling us to temporarily store and manipulate information about potential future actions and their outcomes. Existing research on WM, however, has primarily considered contexts where stimuli are presented simultaneously and encoded independently. It thus remains unclear how WM dynamically manages information about reward and value during planning, when actions are evaluated sequentially in time and their cumulative values must be integrated to guide choice. To address this gap, we developed an information-theoretic model of WM allocation during planning, implemented using variational recurrent neural networks. In this model, an agent optimizes plan quality while maintaining reward information under WM constraints. To test our model, we designed a task in which participants sequentially observed the rewards available at different future states before executing a sequence of actions, attempting to maximize cumulative rewards. Our results suggest that humans preferentially maintain rewards that are most informative for plan selection, integrating both local and global factors. These findings bridge theories of WM limitations with models of human planning, revealing how cognitive constraints shape decision-making strategies.